如果你也在 怎样代写信息论information theory 这个学科遇到相关的难题,请随时右上角联系我们的24/7代写客服。信息论information theory回答了通信理论中的两个基本问题:什么是最终的数据压缩(答案:熵$H$),什么是通信的最终传输速率(答案:信道容量$C$)。由于这个原因,一些人认为信息论是通信理论的一个子集。我们认为它远不止于此。
信息论information theory在统计物理学(热力学)、计算机科学(柯尔莫哥洛夫复杂性或算法复杂性)、统计推断(奥卡姆剃刀:“最简单的解释是最好的”)以及概率和统计学(最优假设检验和估计的误差指数)方面都做出了根本性的贡献。
couryes-lab™ 为您的留学生涯保驾护航 在代写信息论information theory方面已经树立了自己的口碑, 保证靠谱, 高质且原创的统计Statistics代写服务。我们的专家在代写信息论information theory代写方面经验极为丰富,各种代写信息论information theory相关的作业也就用不着说。

数学代写|信息论代写information theory代考|Liquid Water
Water is known to be a structured liquid. However, there is no agreement on how to define the structure of water, see Ben-Naim [9, 10]. Look at Table 2.1, the entropy of vaporization of water is larger than the value expected from Trouton’s rule. Also, we see that the entropy of vaporization of heavy water $\left(\mathrm{D}_2 \mathrm{O}\right)$ is slightly larger than water $\left(\mathrm{H}_2 \mathrm{O}\right)$. This is consistent with the common view that heavy water is a more structured liquid than water. However, we can see in Table 2.1 that ethanol has almost the same entropy of vaporization as heavy water though it is difficult to claim that ethanol is more structured than either water or heavy water.
Tables of standard entropy are available for many liquids as well as for water and heavy water. It is not easy to compare values of standard entropies of different substances with different degrees of freedom such as vibration, rotation and electronic.
In this chapter we have interpreted the entropy values of a simple liquid in terms of the MI associated with the correlation functions which in turn is associated with the strength of the molecular interactions.
It is usually assumes that $\mathrm{H}_2 \mathrm{O}$ and $\mathrm{D}_2 \mathrm{O}$ have approximately the same internal degrees of freedom. It follows that the higher the entropy of vaporization of $\mathrm{D}_2 \mathrm{O}$ compared with $\mathrm{H}_2 \mathrm{O}$ is due to stronger intermolecular interactions. In this case the main part of the interactions is due to hydrogen bonding, see Ben-Naim [9, 10].
Another measure of the “structure” or the extent of intermolecular interactions in the liquid is the entropy of solvation. The solvation process is depicted in Fig. 2.10. A single solute molecule $(s)$ is transferred from a fixed position in an ideal gas phase into a fixed position in an ideal gas phase. Figure 2.11 shows some values of the self-solvation entropy of $\mathrm{H}_2 \mathrm{O}$ and $\mathrm{D}_2 \mathrm{O}$ at several temperatures (self-solvation is the process of solvation of a molecule in its own liquid). In all cases we see that $\Delta S^*$ of $\mathrm{D}_2 \mathrm{O}$ is more negative than the corresponding value of $\mathrm{H}_2 \mathrm{O}$.
It is tradition to interpret these values in terms of structural effects (or ordering). Within our interpretation of entropy as a special case of SMI we view the difference in the values of $\Delta S^*$ in $\mathrm{H}_2 \mathrm{O}$ and $\mathrm{D}_2 \mathrm{O}$ due to the stronger interaction between $\mathrm{D}_2 \mathrm{O}$ molecules compared with $\mathrm{H}_2 \mathrm{O}$ molecules.
In Appendix, we derive a relationship between the entropy of solvation of a solute $s$ in a solvent in terms of difference in SMI. In the next section we also discuss the solvation entropy of inert gases in water. Here however, we discuss the solvation entropy of $\mathrm{H}_2 \mathrm{O}$ in pure $\mathrm{H}_2 \mathrm{O}$, i.e. the “solute” is also a water molecule. This is sometimes called self-solvation, i.e. in Fig. 2.10 instead of a solute $s$ inserted in water, we insert a water molecule into pure water. If we do this process at constant temperature $T$ and volume $V$, the solvation entropy energy is given by:
$$
\Delta S_w^*=\left(k_B \ln 2\right)\left[\operatorname{SMI}\left(N \mid R_s\right)-\operatorname{SMI}(N)\right]
$$
数学代写|信息论代写information theory代考|Aqueous Solutions of Inert Gases
The thermodynamics of aqueous solutions of inert gases involves a few, very exciting and mysterious problems. We shall discuss in this section only one aspect of these systems; the solvation entropy of an inert solute, say, argon in water.
The solubilities of inert solutes such as argon, neon, methane and the like are very small. In the early 1930 s and 1940 s the data available on the solubility of these solutes in water was very inaccurate. It was known that the solubility of these solutes in water is much smaller than in other organic liquid.
The entropy of solvation (previously referred to as the entropy of solution) of these solutes could be obtained only from very accurate data on the solubility and its dependence on temperature. For more details, see Ben-Naim [11, 12]. It was known that the entropy of solvation of inert solutes in water is large and negative compared with the solvation entropy of the same solutes in typical organic solvents. A few examples are shown in Table 2.2.
In 1945, Frank and Evans [13] published a very influential article on the thermodynamics of solvation of inert solutes in water and in other liquids. They noted that the entropy of solvation of these solutes is much larger and negative in water as compared with the entropy of solvation of the same solutes in other liquids. To explain these findings, the authors conjectured that when an inert solute dissolves in water it forms, or builds some kind of structure, which the called “icebergs,” around it. This idea was revolutionary at that time. It has captured the imagination of many scientists for more than half a century. How can an inert solute, weakly interacting with water molecules, form an “iceberg”? Frank and Evans did not offer any proof that an inert solute builds up iceberg around it, nor did they provide any explanation as to why inert solute should form icebergs. All they did was to interpret the negative change in entropy in terms of increasing the order, or equivalently increasing the structure of water. Yet, this idea was not only accepted by, but used by many scientists to explain the entropy and the enthalpy of solvation of the non-polar solute in water.
The truth is that Frank and Evans did not contribute anything to understanding the entropy of solvation of inert gases in water. The last statement might be shocking to many chemists who believe that Frank and Evans actually solved the problem. Unfortunately, they did not. Entropy at that time was viewed (and still is) as a measure of the extent of order or disorder in the system. “Structure” is another word for order. Therefore, Frank and Evans suggestion was nothing but the translation of the experiment fact about the negative entropy of solvation into the language of order-disorder. Thus, negative $\Delta S_s^*$ is equivalent to more order, or more structure, or picturesquely formation of icebergs. For more details, see Ben-Naim [9].

信息论代写
数学代写|信息论代写information theory代考|Liquid Water
众所周知,水是一种结构液体。然而,关于如何定义水的结构尚无一致意见,参见Ben-Naim[9,10]。从表2.1可以看出,水的蒸发熵大于Trouton规则的期望值。同时,我们看到重水的蒸发熵$\left(\mathrm{D}_2 \mathrm{O}\right)$略大于水$\left(\mathrm{H}_2 \mathrm{O}\right)$。这与一般认为重水是比水更有结构的液体的观点是一致的。然而,从表2.1中我们可以看出,乙醇的蒸发熵与重水几乎相同,尽管很难断言乙醇比水或重水更有结构。
标准熵表不仅适用于水和重水,也适用于许多液体。振动、旋转、电子等不同自由度物质的标准熵值比较不容易。
在本章中,我们用与相关函数相关的熵值来解释简单液体的熵值,而相关函数又与分子相互作用的强度相关。
通常假设$\mathrm{H}_2 \mathrm{O}$和$\mathrm{D}_2 \mathrm{O}$具有近似相同的内部自由度。由此可见,与$\mathrm{H}_2 \mathrm{O}$相比,$\mathrm{D}_2 \mathrm{O}$的汽化熵越高,是由于分子间的相互作用越强。在这种情况下,相互作用的主要部分是由于氢键,见Ben-Naim[9,10]。
液体中分子间相互作用的“结构”或程度的另一个量度是溶剂化熵。溶剂化过程如图2.10所示。单个溶质分子$(s)$从理想气相中固定的位置转移到理想气相中固定的位置。图2.11给出了$\mathrm{H}_2 \mathrm{O}$和$\mathrm{D}_2 \mathrm{O}$在不同温度下的一些自溶剂化熵值(自溶剂化是分子在其自身液体中的溶剂化过程)。在所有情况下,我们看到$\mathrm{D}_2 \mathrm{O}$的$\Delta S^*$比$\mathrm{H}_2 \mathrm{O}$的相应值更负。
传统上用结构效应(或顺序)来解释这些价值。在我们将熵解释为SMI的特殊情况时,我们认为$\mathrm{H}_2 \mathrm{O}$和$\mathrm{D}_2 \mathrm{O}$中$\Delta S^*$值的差异是由于$\mathrm{D}_2 \mathrm{O}$分子与$\mathrm{H}_2 \mathrm{O}$分子之间的相互作用更强。
在附录中,我们推导了溶剂中溶质$s$的溶剂化熵与SMI差异之间的关系。下一节我们还将讨论惰性气体在水中的溶剂化熵。然而,这里我们讨论的是$\mathrm{H}_2 \mathrm{O}$在纯$\mathrm{H}_2 \mathrm{O}$中的溶剂化熵,即“溶质”也是一个水分子。这有时被称为自溶剂化,即在图2.10中,我们将水分子插入纯水中,而不是将溶质$s$插入水中。如果我们在恒定温度$T$和体积$V$下进行这个过程,溶剂化熵能为:
$$
\Delta S_w^*=\left(k_B \ln 2\right)\left[\operatorname{SMI}\left(N \mid R_s\right)-\operatorname{SMI}(N)\right]
$$
数学代写|信息论代写information theory代考|Aqueous Solutions of Inert Gases
惰性气体水溶液的热力学涉及几个非常令人兴奋和神秘的问题。本节我们将只讨论这些系统的一个方面;惰性溶质的溶剂化熵,例如氩气在水中的溶剂化熵。
惰性溶质如氩、氖、甲烷等溶解度很小。在20世纪30年代和40年代早期,关于这些溶质在水中溶解度的可用数据是非常不准确的。已知这些溶质在水中的溶解度比在其他有机液体中的溶解度小得多。
这些溶质的溶剂化熵(以前称为溶液熵)只能从溶解度及其对温度的依赖关系的非常精确的数据中得到。更多细节请参见Ben-Naim[11,12]。已知惰性溶质在水中的溶剂化熵比相同溶质在典型有机溶剂中的溶剂化熵大且为负。表2.2给出了几个例子。
1945年,Frank和Evans[13]发表了一篇非常有影响力的关于惰性溶质在水和其他液体中的溶剂化热力学的文章。他们注意到,与相同溶质在其他液体中的溶剂化熵相比,这些溶质在水中的溶剂化熵要大得多,而且是负的。为了解释这些发现,作者推测,当惰性溶质溶解在水中时,它会在其周围形成或形成某种结构,即所谓的“冰山”。这个想法在当时是革命性的。半个多世纪以来,它俘获了许多科学家的想象力。一个与水分子弱相互作用的惰性溶质是如何形成“冰山”的?弗兰克和埃文斯没有提供任何证据证明惰性溶质在其周围形成冰山,也没有提供任何解释为什么惰性溶质会形成冰山。他们所做的就是把熵的负变化解释为序的增加,或者等价地说,水的结构的增加。然而,这个想法不仅被许多科学家所接受,而且还被许多科学家用来解释水中非极性溶质的熵和溶剂化焓。
事实是,弗兰克和埃文斯对理解惰性气体在水中的溶剂化熵没有任何贡献。最后一句话可能会让许多化学家感到震惊,他们相信弗兰克和埃文斯实际上解决了这个问题。不幸的是,他们没有。熵在当时被视为(现在仍然是)衡量系统中有序或无序程度的尺度。“结构”是秩序的另一个词。因此,Frank和Evans的建议只不过是将溶剂化负熵的实验事实翻译成有序-无序的语言。因此,负的$\ δ S_s^*$等价于更多的秩序,或更多的结构,或冰山如画般的形成。更多细节请参见Ben-Naim[9]。
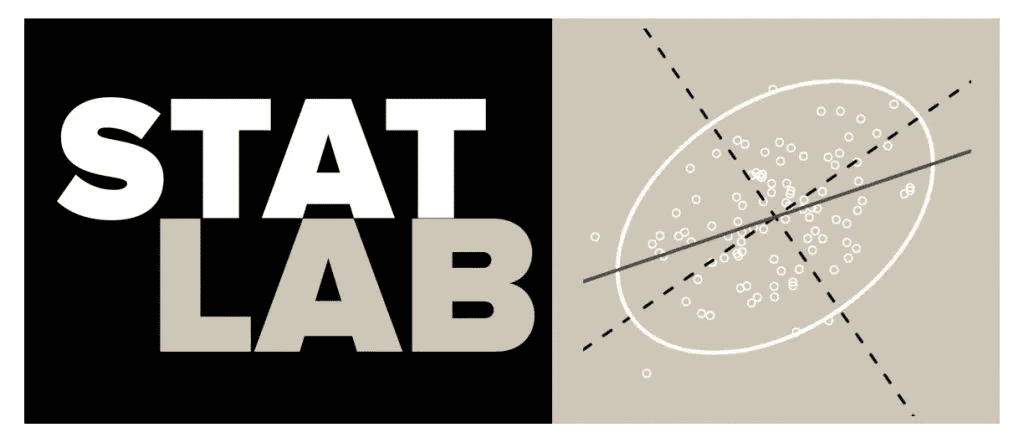
统计代写请认准statistics-lab™. statistics-lab™为您的留学生涯保驾护航。
金融工程代写
金融工程是使用数学技术来解决金融问题。金融工程使用计算机科学、统计学、经济学和应用数学领域的工具和知识来解决当前的金融问题,以及设计新的和创新的金融产品。
非参数统计代写
非参数统计指的是一种统计方法,其中不假设数据来自于由少数参数决定的规定模型;这种模型的例子包括正态分布模型和线性回归模型。
广义线性模型代考
广义线性模型(GLM)归属统计学领域,是一种应用灵活的线性回归模型。该模型允许因变量的偏差分布有除了正态分布之外的其它分布。
术语 广义线性模型(GLM)通常是指给定连续和/或分类预测因素的连续响应变量的常规线性回归模型。它包括多元线性回归,以及方差分析和方差分析(仅含固定效应)。
有限元方法代写
有限元方法(FEM)是一种流行的方法,用于数值解决工程和数学建模中出现的微分方程。典型的问题领域包括结构分析、传热、流体流动、质量运输和电磁势等传统领域。
有限元是一种通用的数值方法,用于解决两个或三个空间变量的偏微分方程(即一些边界值问题)。为了解决一个问题,有限元将一个大系统细分为更小、更简单的部分,称为有限元。这是通过在空间维度上的特定空间离散化来实现的,它是通过构建对象的网格来实现的:用于求解的数值域,它有有限数量的点。边界值问题的有限元方法表述最终导致一个代数方程组。该方法在域上对未知函数进行逼近。[1] 然后将模拟这些有限元的简单方程组合成一个更大的方程系统,以模拟整个问题。然后,有限元通过变化微积分使相关的误差函数最小化来逼近一个解决方案。
tatistics-lab作为专业的留学生服务机构,多年来已为美国、英国、加拿大、澳洲等留学热门地的学生提供专业的学术服务,包括但不限于Essay代写,Assignment代写,Dissertation代写,Report代写,小组作业代写,Proposal代写,Paper代写,Presentation代写,计算机作业代写,论文修改和润色,网课代做,exam代考等等。写作范围涵盖高中,本科,研究生等海外留学全阶段,辐射金融,经济学,会计学,审计学,管理学等全球99%专业科目。写作团队既有专业英语母语作者,也有海外名校硕博留学生,每位写作老师都拥有过硬的语言能力,专业的学科背景和学术写作经验。我们承诺100%原创,100%专业,100%准时,100%满意。
随机分析代写
随机微积分是数学的一个分支,对随机过程进行操作。它允许为随机过程的积分定义一个关于随机过程的一致的积分理论。这个领域是由日本数学家伊藤清在第二次世界大战期间创建并开始的。
时间序列分析代写
随机过程,是依赖于参数的一组随机变量的全体,参数通常是时间。 随机变量是随机现象的数量表现,其时间序列是一组按照时间发生先后顺序进行排列的数据点序列。通常一组时间序列的时间间隔为一恒定值(如1秒,5分钟,12小时,7天,1年),因此时间序列可以作为离散时间数据进行分析处理。研究时间序列数据的意义在于现实中,往往需要研究某个事物其随时间发展变化的规律。这就需要通过研究该事物过去发展的历史记录,以得到其自身发展的规律。
回归分析代写
多元回归分析渐进(Multiple Regression Analysis Asymptotics)属于计量经济学领域,主要是一种数学上的统计分析方法,可以分析复杂情况下各影响因素的数学关系,在自然科学、社会和经济学等多个领域内应用广泛。
MATLAB代写
MATLAB 是一种用于技术计算的高性能语言。它将计算、可视化和编程集成在一个易于使用的环境中,其中问题和解决方案以熟悉的数学符号表示。典型用途包括:数学和计算算法开发建模、仿真和原型制作数据分析、探索和可视化科学和工程图形应用程序开发,包括图形用户界面构建MATLAB 是一个交互式系统,其基本数据元素是一个不需要维度的数组。这使您可以解决许多技术计算问题,尤其是那些具有矩阵和向量公式的问题,而只需用 C 或 Fortran 等标量非交互式语言编写程序所需的时间的一小部分。MATLAB 名称代表矩阵实验室。MATLAB 最初的编写目的是提供对由 LINPACK 和 EISPACK 项目开发的矩阵软件的轻松访问,这两个项目共同代表了矩阵计算软件的最新技术。MATLAB 经过多年的发展,得到了许多用户的投入。在大学环境中,它是数学、工程和科学入门和高级课程的标准教学工具。在工业领域,MATLAB 是高效研究、开发和分析的首选工具。MATLAB 具有一系列称为工具箱的特定于应用程序的解决方案。对于大多数 MATLAB 用户来说非常重要,工具箱允许您学习和应用专业技术。工具箱是 MATLAB 函数(M 文件)的综合集合,可扩展 MATLAB 环境以解决特定类别的问题。可用工具箱的领域包括信号处理、控制系统、神经网络、模糊逻辑、小波、仿真等。